Both present and future
Artificial intelligence is already an integral part of many applications. Researchers and developers are now working hard to find better ways of getting more out of foundation models.
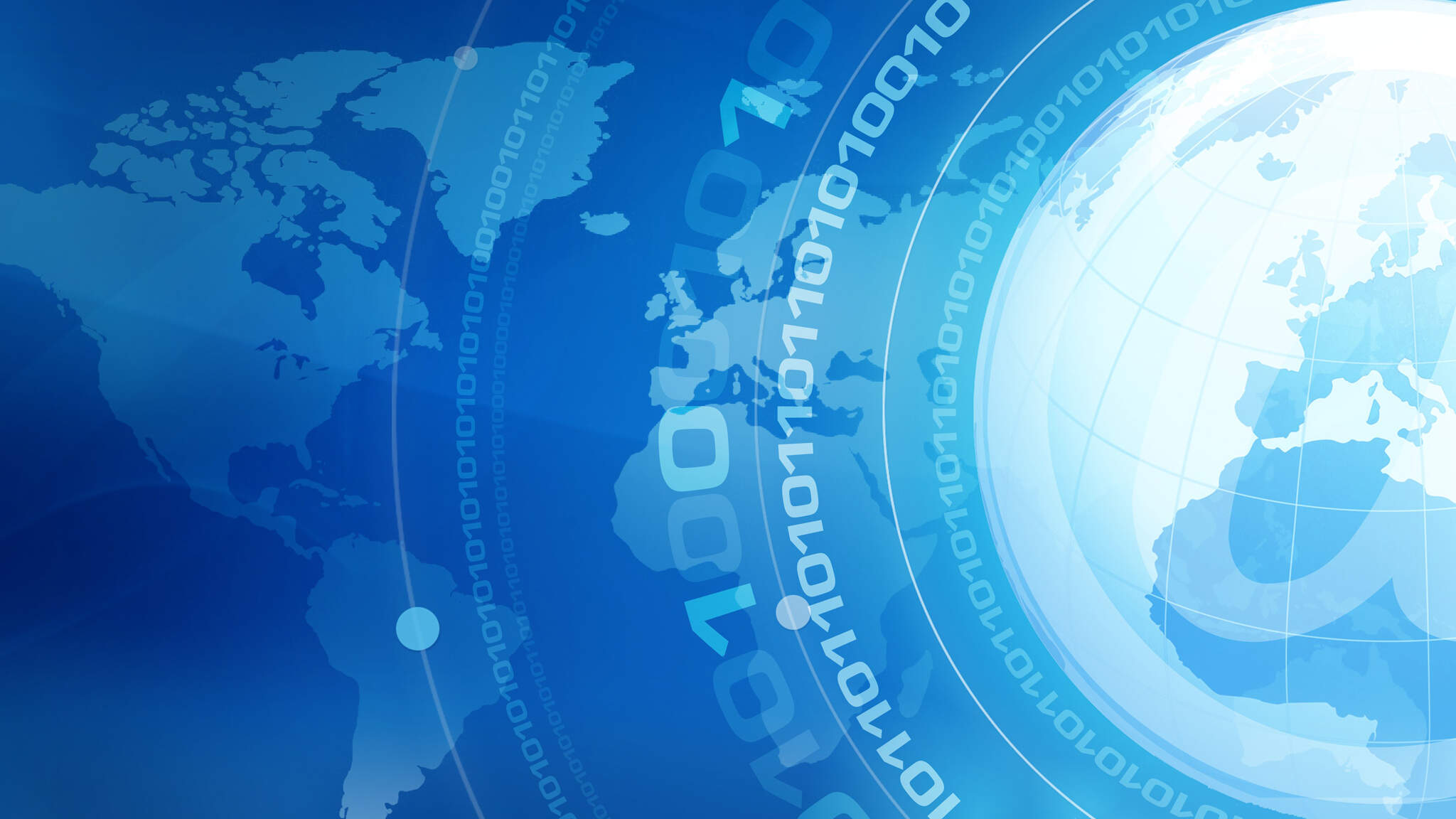
Is artificial intelligence (AI) a technology of the distant future? The answer is both yes and no. No, because AI applications have long since become a part of our everyday lives. Take facial recognition, which many people use to unlock their smartphones. Or translation services that are now highly adept at accurately rendering phrases and even entire documents in a host of other languages in a matter of seconds. Then there are chatbot systems like ChatGPT and Copilot, which provide today’s school and university students with a whole new way of writing papers.
These are three examples of IT applications that are not based on traditional if-then programming. Instead, they use vast amounts of training data, advanced learning methods such as supervised or reinforcement learning, and algorithms that often employ highly complex neural networks. An AI algorithm will never produce “0” or “1” as a result. Instead, the AI always provides the probability of a mathematically calculated prediction being correct, and this will never be 100 percent. Assertions made by artificial intelligence are always at least a little off. And it’s precisely this characteristic that requires results to be scrutinized—although this in no way negates the technology’s vast potential. Indeed, AI is the only way to calculate practicable solutions for the most complex correlations. AI applications are rather like a smart colleague who has a lot of knowledge, but is occasionally mistaken.
AI has also arrived in logistics. More than six years ago, the team at the DACHSER Enterprise Lab at Fraunhofer IML began developing algorithms to forecast tonnage volumes for DACHSER’s groupage network 25 weeks in advance. They also came up with an image recognition solution to identify, locate, and measure packages in groupage warehouses in real time. For several years, the cornerstone of DACHSER’s AI implementation strategy has been to have its logistics specialists and process experts collaborate with mathematicians and software developers.
New, unexpected possibilities
Nevertheless, artificial intelligence can also still be considered a technology of the future. New models continue to open up unexpected possibilities. At the forefront here are the foundation models for generative AI, which use advanced algorithms, trained on masses of data culled from the internet, to understand and create texts and images. ChatGPT and other large language models (LLMs) in particular give the impression of possessing “intelligence.” However, this is really just based on a mathematical function that predicts a coherent word order.
Foundation models in AI: In the field of artificial intelligence (AI), a foundation model is a large, pretrained model based on vast datasets that is compatible with a wide range of applications. There are different types of foundation models, including large language models (LLMs) and visual processing models. LLMs such as GPT-4 from OpenAI, Gemini (formerly “Bard”) and Bert from Google, and Llama3 from Meta specialize in understanding and generating natural language. Visual models such as Sora and DALL-E from OpenAI are designed to generate videos and images using free-form text input (prompts). As the name suggests, foundation models often serve as the basis for specialized applications, for which they are adapted to incorporate specific tasks or datasets.
But there’s no getting around the fact that these models produce impressive results, and we have only just begun to tap their vast potential. This would enhance robots’ ability to perform complex tasks such as natural language processing, image and object recognition, and autonomous navigation. These models allow robots to learn from vast amounts of data and adapt to new environments and tasks, which in means they offer greater flexibility and in a wider range of applications. And it won’t be long before we see if the autonomous vehicles used in warehouses can be controlled more intuitively and efficiently. Intensive research is being carried out worldwide.
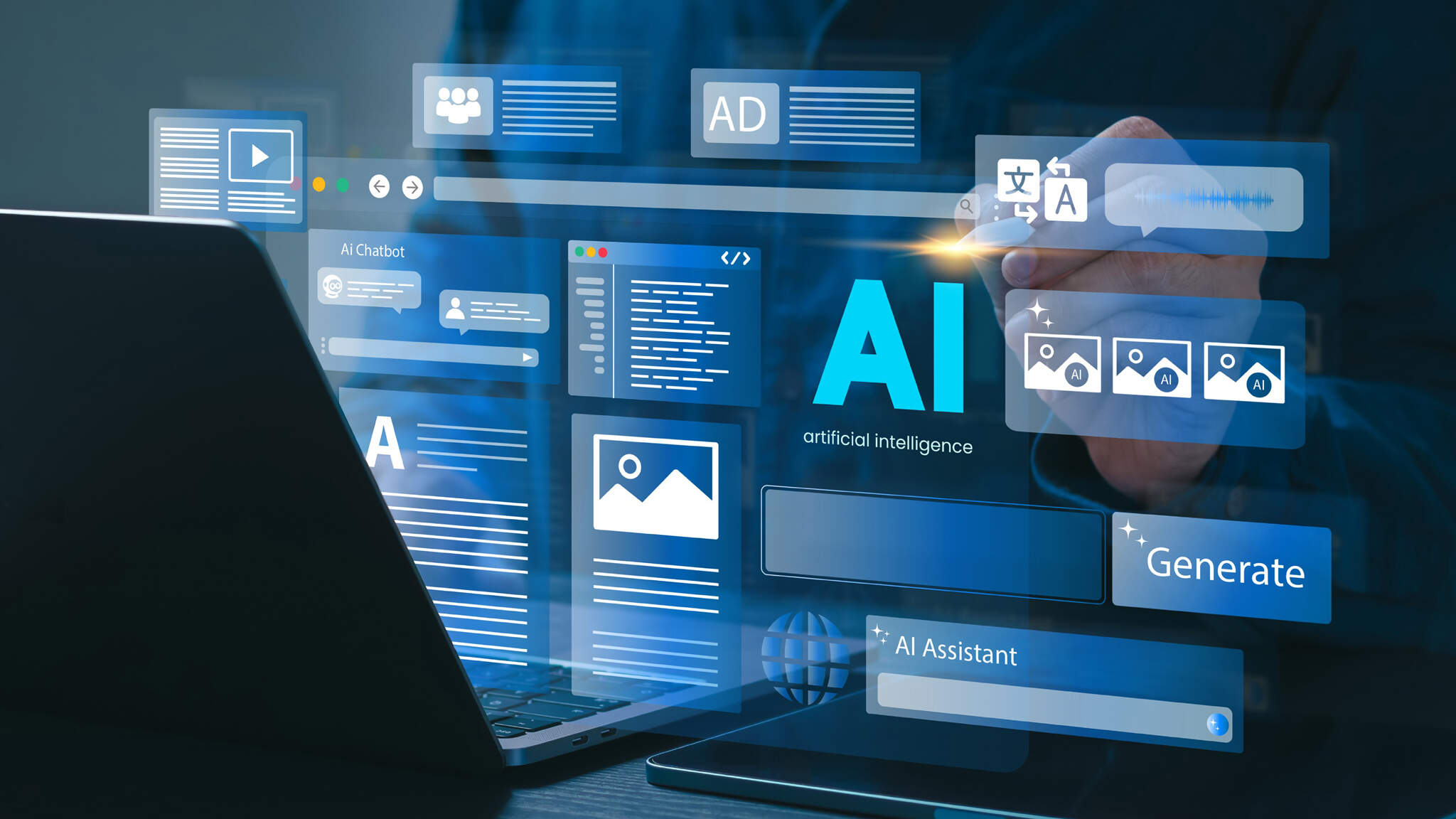
RAG: A better basis for AI-assisted research
Many developments in AI focus on retrieval-augmented generation (RAG), which promises to enhance the quality of the results produced by large foundation models. Essentially, RAG furnishes LLMs with higher-quality data and knowledge sources for the given use case. This prevents the LLM from fabricating results should it be unable to come up with a solution that has a high probability of being correct. Such misbehavior in LLM tools is referred to as a “hallucination” and can often undermine users’ trust in artificial intelligence.
Further research into artificial intelligence will yield a whole new range of potential applications. Companies like DACHSER have to find the right mix between the use of standardized AI applications and in-house developments. AI models must be trained with specific internal company data, especially for special logistics processes and solutions. The general information available on the internet is not sufficient as a basis for training. At the same time, consideration must be given to the costs, especially of AI models that require considerable computing power, as well as to compliance with the EU’s new legal framework for AI applications as laid out in the AI Act. Both industry and society are only just beginning to delve deeper into using artificial intelligence—a journey that will surely present its fair share of challenges.
Author: Andre Kranke, Head of Corporate Research & Development at DACHSER