Machine learning – Support from the data cosmos
Digitalization makes work in logistics easier and more efficient. Goods and data streams flow together, creating quality and transparency across all process steps. DACHSER can apply machine learning to analyze and use data from day-to-day operations, opening up new horizons for intelligent logistics solutions that add value.
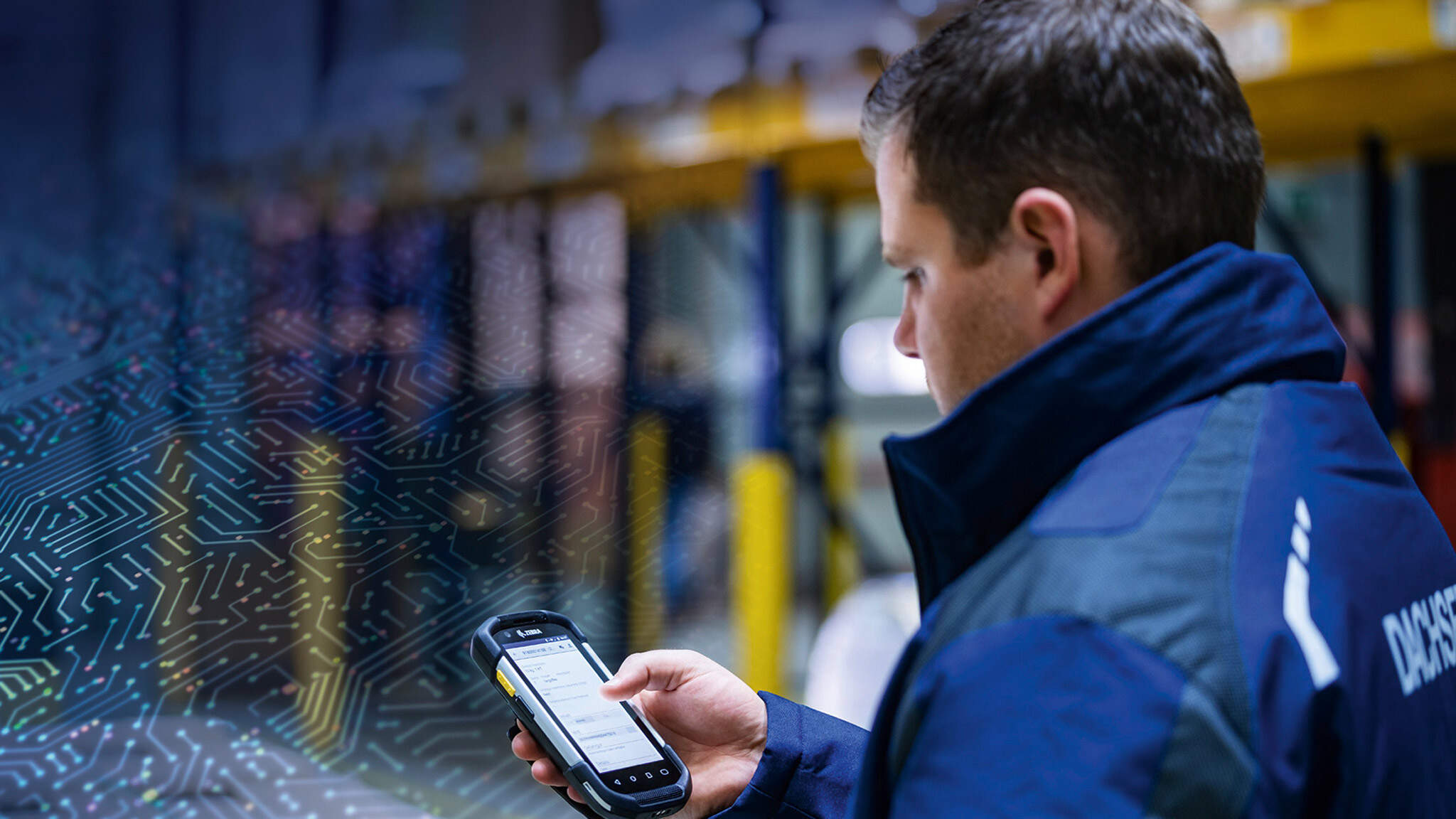
“Data is the oil of the 21st century.” The British mathematician and data scientist Clive Humby is said to have first made this analogy back in 2006 when developing a loyalty card. At the time, it was a visionary claim. Today, Humby’s vision has long since become an everyday reality, and even politicians pick up on the image again and again when they call for progress. And with good reason: every aspect of our lives is embedded in an ever-growing stream of data that is swelling exponentially. This data stream is just as much a part of our reality as a quick glance at the smartphone, chats with friends and family, or video conferences in day-to-day business.
Data has become a decisive economic factor, and the “Big Five” tech companies—Google, Apple, Facebook, Amazon, and Microsoft—are the Rockefellers and oil sheikhs of the 21st century. They have long since overtaken the oil supermajors as the most valuable companies in the world.
Some observers are uneasy about this expansion of data dominance; they paint gloomy pictures of companies scooping up our data and the rapid decline of data protection and personal rights. But the benefits ultimately outweigh the reservations as life becomes easier and less complicated, whether in online interactions across national and cultural borders or in the real-time availability of news and knowledge content, music and literature, and up-to-the-minute navigation tools for avoiding traffic and congestion.
Boosting efficiency with data analysis
In the manufacturing and service sectors, data drives significant efficiency gains. For instance, data analysis makes it possible to better monitor operational processes, detect and eliminate errors and inefficiencies at an early stage, and continuously optimize procedures. In logistics, data and data analysis have long been an integral part of the management of goods and supply chains. They are the basis for reliable logistics processes designed for maximum transparency, be it in putting together loads, planning transport routes, combining different means of transport, or tracking shipments—in short, management of the en-tire network.
“The importance of artificial intelligence, machine learning, and data science for transportation, logistics, and supply chain management will continue to grow in the coming years.”
DACHSER and its global networks have already “learned” all of this. As early as the mid-1980s, the logistics company developed Domino, the cornerstone of its concept for processing freight-forwarding data. The software covers all processes related to the transport of goods—not just outbound and inbound in the transit terminal, or import and export, but also order administration, transport scheduling, billing, and above all, shipment information: tracking and tracing. Today, in addition to Domino, DACHSER has two other deeply integrated systems: Mikado (warehouse) and Othello (air and sea freight), both of which it continuously expands. The B2B Gateway serves as the central communication platform for all of them. Meanwhile, DACHSER customers can manage orders online using the eLogistics portal. Added to this is the ActiveReport supply chain event management tool, which sounds the alarm when discrepancies arise in the shipment process. In short: DACHSER and its customers are at home in the world of data and make use of it for fulfillment every day.
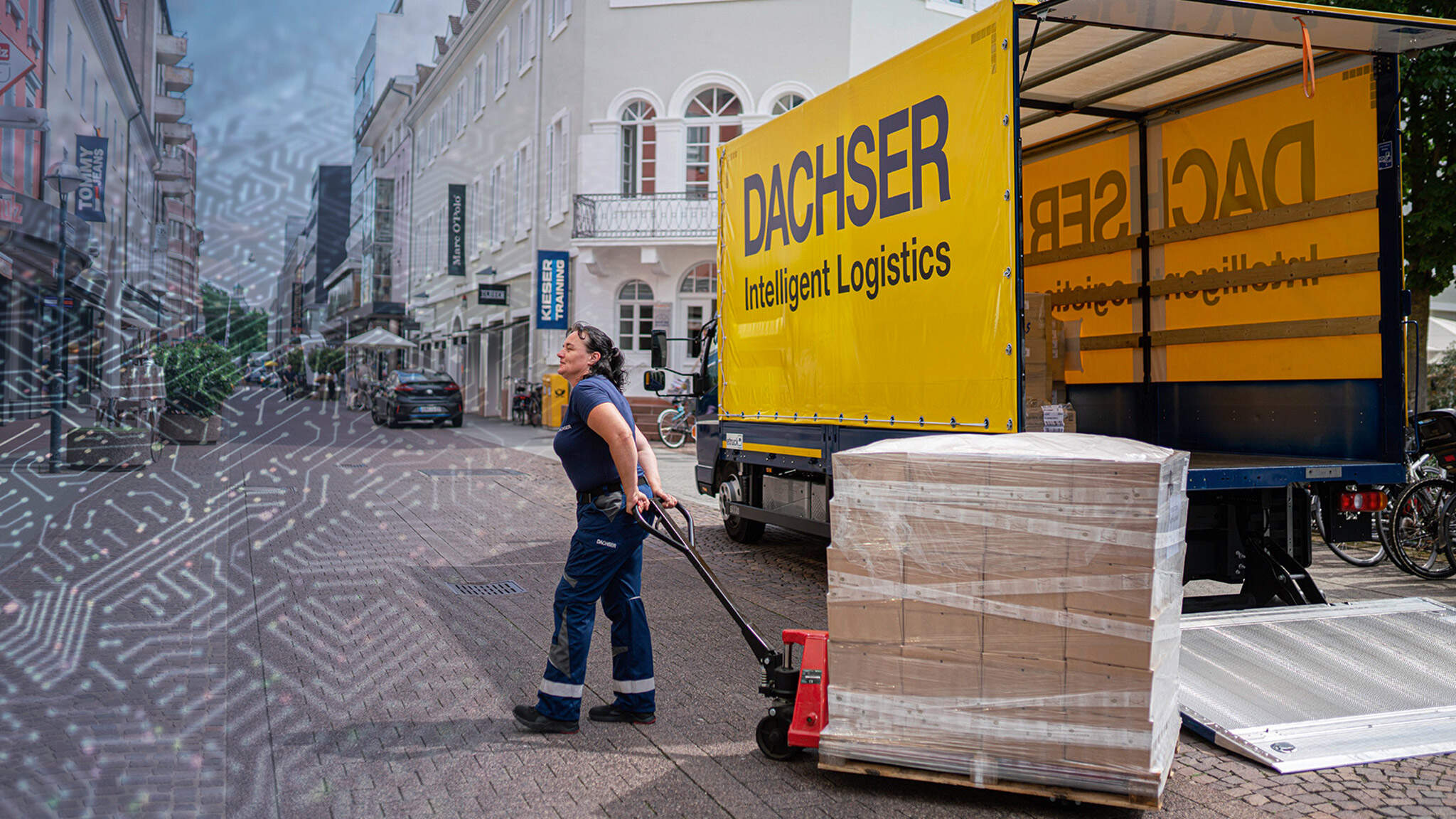
You can’t go it alone
To fully tap the benefits of digitalization and further advance network maturity, DACHSER is cultivating an even deeper fusion of IT and logistics. “Here we need freight forwarding experts and logistics practitioners just as much as statisticians, mathematicians, and computer scientists. You can’t get there on your own—the only way to develop intelligent algorithms for logistics is by teaming up with others,” notes Stefan Hohm, Chief Development Officer (CDO) at DACHSER. He explains that this is an ongoing process that not only reflects technological development, but also helps drive it.
Since the beginning of June 2021, DACHSER has pooled the expertise it has gained in various research and innovation projects in its new in-house competence center for data science and machine learning.
“The importance of artificial intelligence, machine learning, and data science for transportation, logistics, and supply chain management will continue to grow in the coming years. That makes it crucial for DACHSER to further strengthen its expertise in this important field and to expand its ability to implement and operate machine learning applications,” Hohm says.
The background for this pooling of expertise arises from everyday logistics operations. DACHSER produces large volumes of data on a daily basis, and this forms a foundation for the development and use of the new AI technologies. “We will make even better use of this data in the future and provide our employees with an even better basis for decision-making,” says Florian Zizler, Team Leader Data Science & Machine Learning. Mention “artificial intelligence,” however, and what comes to mind isn’t always positive. People think of uncontrollable “big data” machines with a life of their own, of robots replacing humans and eventually making them completely superfluous in the world of work. It helps to first clarify some terms.
Doomed to constant learning
British logician, mathematician, cryptanalyst, and computer scientist Alan Turing (1912–1954), one of the masterminds of modern information and computer technology, made a pragmatic proposal for narrowing down the very broad field of AI: a machine is intelligent if it cannot be determined whether you are communicating with a person or a computer. And Irish dramatist George Bernard Shaw (1856–1950) observed that the disadvantage of intelligence is that you are continuously forced to learn.
In artificial intelligence, it’s the algorithms that do the learning (for those non-mathematicians out there, an algorithm is a sequence of instructions and computational operations within a software program that can be used to solve certain problems). And this is exactly where machine learning comes in as a subdiscipline of AI. By looking at examples, the AI system learns to recognize patterns and regularities in processes and contexts in order to master unknown situations.
DACHSER recently developed concrete machine-learning applications as part of the DACHSER Enterprise Lab together with scientists from the Fraunhofer IML institute in Dortmund, Germany. One such application is PAnDA One: Predictive Analytics DACHSER, where “One” denotes that it is the company’s first machine learning project.
The PAnDA One model was specifically designed to forecast inbound volumes for a Road Logistics branch. “Our goal is to provide employees in our branches with valuable support on decisions they need to make regarding seasonal capacity planning,” explains Thomas Schmalz, Head of Production Management at DACHSER. That makes it possible to obtain appropriate load capacity on the market at an early stage, or to plan resources in the transit terminal. “To that end, the forecasting model provides appropriate inbound volumes up to 25 weeks in advance.”
Machine learning is not an end in itself, Schmalz emphasizes: “We want to give our branches a tool that ultimately makes their work easier, more efficient, and also more attractive.” DACHSER’s goal is not to replace people with calculations and computing. “We view IT, technology, the network, and people as a single unit: a cyber-socio-physical system. This is how we move our customers—and ourselves—forward.”
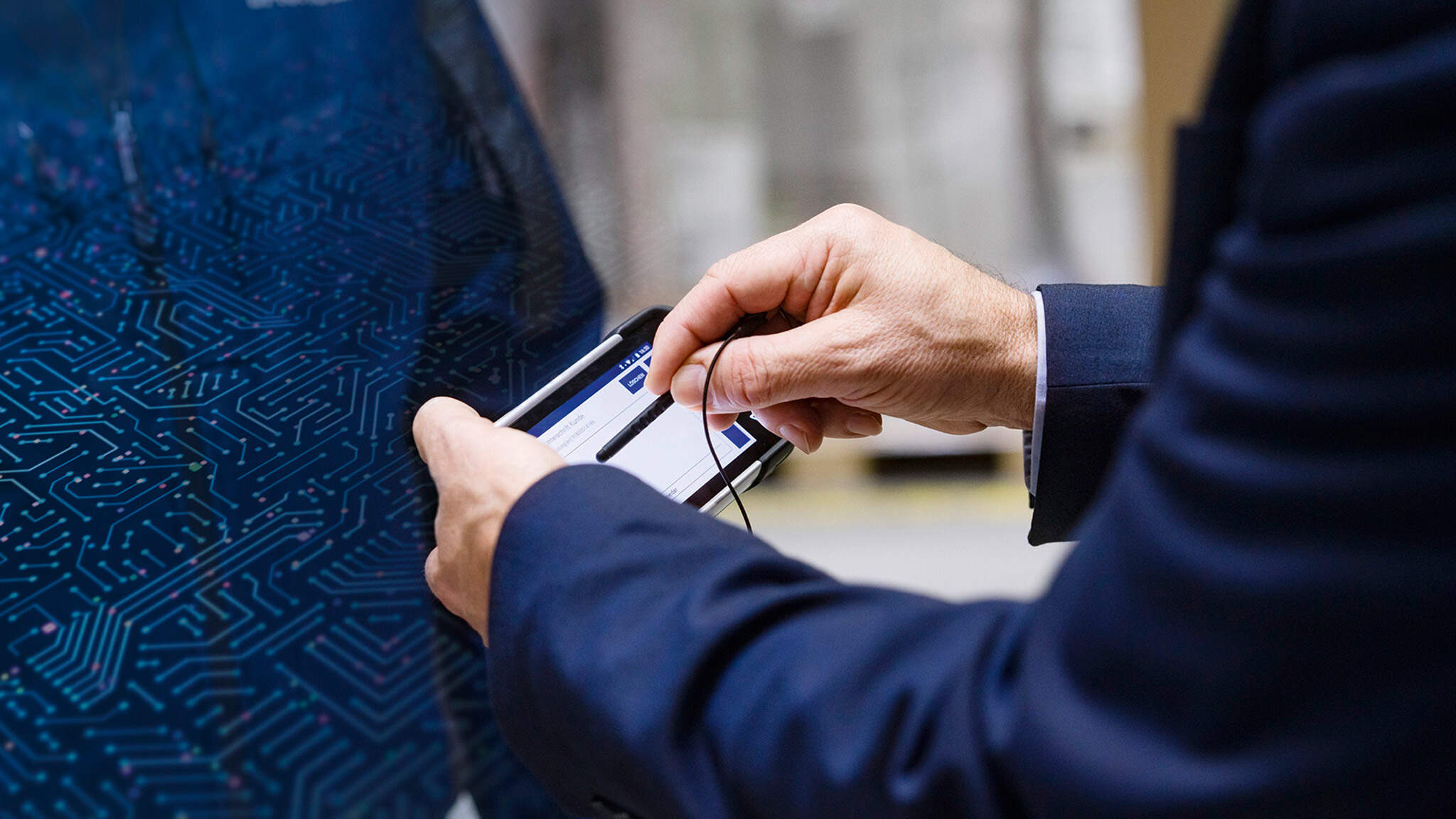
Data quality is everything
The success of machine learning depends on the quality of the input data. “The data itself is more important than the algorithm,” Schmalz says. “We have a unique pool that receives consistent data in assured and standardized quality.” DACHSER has an advantage here because of its uniform system landscape in European Logistics and Air & Sea Logistics and because of its network maturity, which has grown over decades. “There aren’t too many in logistics who can offer that.”
Having good data is one thing, but interpreting and using it is another. “Machine learning is a team effort. Successful models are possible only when process experts work hand in hand with the experts from the Data Science & Machine Learning Competence Center. We need each other,” Zizler says.
DACHSER’s competence center for data science and machine learning connects the worlds of logistics and IT. “We’re creating a space where a wide variety of stakeholders can come together and contribute their subject matter expertise. The exchange is immediate and direct. Ultimately, it’s about using data to model concrete processes,” Zizler says. “It’s a great experience for everyone to approach highly specific tasks in an interdisciplinary exchange and to develop helpful solutions for everyday logistics from them.”
For PAnDA One, the DACHSER experts in the competence center worked with the different departments to comprehensively analyze processes and identify criteria for reliable forecasts. “Our data goes back as far as 2011. The focus is on historical shipment data,” Zizler says. “We supplement this data pool with calendar data, such as public holidays or school vacations. This enables the model to recognize the seasonal patterns that are so important in overland transport. To better anticipate trends, we’ve also integrated a wide variety of economic indices.”
However, the predictability of future requirements and customer needs still reaches its limits when conditions change due to major unforeseen events. “Of course, it was a challenge for forecasts to cope with volatile volume fluctuations as well as the coronavirus pandemic,” Zizler says. But he and his team of experts remain optimistic: “We’ll soon get our forecasting back to its usual high quality.”
Another specific use case is the B2X Labeling project. The job of the algorithm is to improve data quality and solve a classification problem from everyday operations, namely, determining whether the recipient is a company (B2B) or a private individual (B2C). Based on the order data alone, this distinction is not always clear. For logistics processes, however, it makes a considerable difference whether the receiver is a company or a private household.
In machine learning, there is a fundamental distinc-tion between a training phase and an application phase. During training, the algorithm is shown sample data to learn how the input is transformed into the output. Input data for the B2X project focuses on order data such as geographical data. In the subsequent application phase, the trained model uses the learned correlations to generate an output (B2B or B2C) under real operating conditions based on new information. This project plays a vital role in quality assurance by not only increasing data quality, but also further optimizing processes in logistics handling.
The competence center continuously evaluates new ideas and potential use cases. One of these is in process optimization and the improvement of ergonomic working conditions for logistics operatives in cargo handling. Here, machine learning experts are testing a new process for boosting process quality together with the start-up MotionMiners at five locations (we reported on this in DACHSER magazine 2/2021).
The range of applications for machine learning is broad. In the course of exploring them, a robust and dynamic open source ecosystem has developed in recent years. “Machine learning will increasingly shape DACHSER’s diverse process landscape. Thanks to the availability of open source applications, we no longer have to develop everything ourselves. Skillful use of these algorithms will let us tap our data potential even more effectively,” Zizler says. This is one of the reasons why DACHSER is a founding member of the Open Logistics Foundation (more on this on page 30). This is, then, another difference from the oil of past centuries: data doesn’t run out. Quite the opposite, in fact! An excellent foundation for the future.